In the complex and ever-evolving landscape of vehicle insurance, an alarming statistic stands out: 20% of drivers are thought to be either underinsured or uninsured, revealing a crucial gap in risk management and coverage. This obvious problem not only emphasizes how difficult it is to evaluate and price insurance policies effectively, but it also shows how ineffective standard models are at meeting the various needs and risk profiles of drivers. These inefficiencies present a strong argument for the insurance sector to look for novel solutions, especially in light of the quick advances in technology and customer expectations. Among these, the incorporation of data science stands out as a viable avenue that could transform the way insurance businesses see, interact with, and serve their clientele.
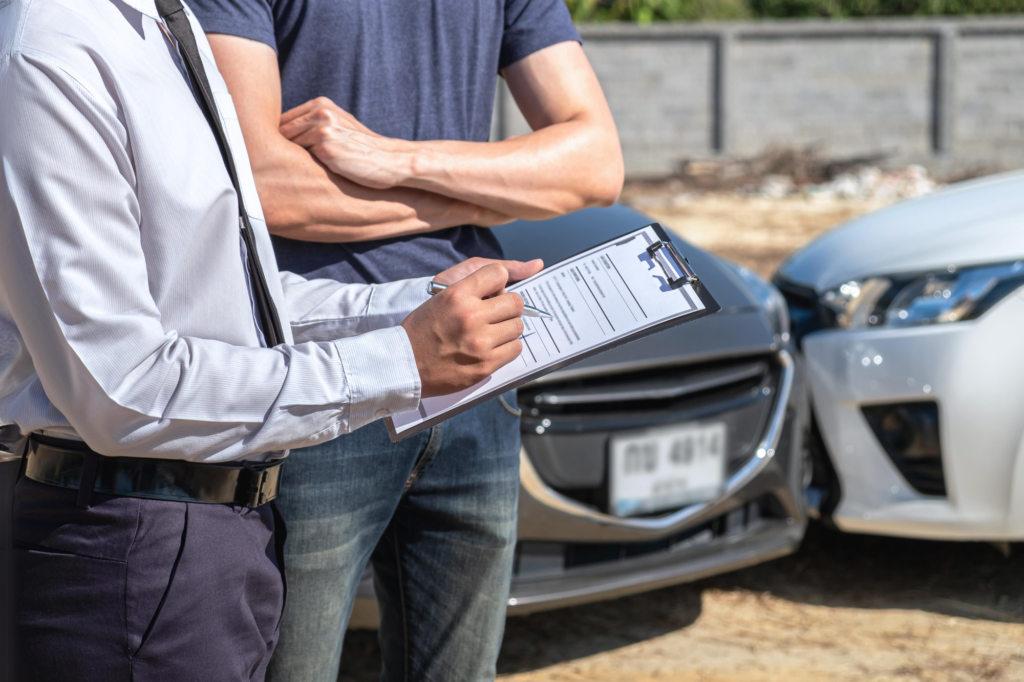
The application of data science has the potential to completely change the way auto insurance firms target their customers and set their prices. Insurers can gain a more comprehensive picture of risk profiles by utilizing sophisticated analytics and machine learning to examine behavioral factors such as driving habits, health routines, and lifestyle choices[1]. More accurate and equitable pricing is ensured by this granular research, which enables dynamic premium quoting that closely matches the risk profiles and price sensitivities of each individual customer [2].
Data science also makes more specialized coverage alternatives possible, guaranteeing that clients obtain policies that are precisely tailored to their individual requirements and risk profiles. Through the provision of pertinent and reasonably priced plans, this personalization raises customer satisfaction and encourages increased loyalty and faith in the insurance company [3].
More broadly, insurance businesses can reduce overhead expenses and increase their bottom line by streamlining operations, automating underwriting decisions, and proactively identifying possible hazards thanks to the efficiency obtained by data-driven insights [4]. As a result, the application of data science to auto insurance improves customer targeting and pricing while also driving businesses toward increased productivity, client happiness, and profitability.
Section 1: The Current Landscape of Vehicle Insurance
Overview of Traditional Practices :
Traditionally, vehicle insurance companies have relied on actuarial science to assess risk and determine pricing. This entails utilizing rate tables that are calculated by actuaries and account for a number of variables, including the age of the driver, the vehicle’s type, planned use, and driving history [5]. The insurance prices are determined by taking into account many factors such as the driver’s credit score, geography, and demographics in some states [6].
limitations :
These conventional methods do have some serious drawbacks, though. A common consequence of the broad classification of risk factors is that insurance plans become less customized. For instance, even when one driver has a substantially better driving record than the other, two drivers who are the same age and drive the same model of car can be assessed comparable premiums. Because of this lack of detail, premiums may be determined inaccurately, potentially resulting in lower-risk drivers footing the bill for higher-risk drivers. Moreover, conventional approaches might not adequately take into consideration the subtleties of each person’s risk profile or the intricacies of contemporary driving behavior, which could result in inefficiencies in coverage and price [7], [8].
Section 2: The Potential of Data Science in Insurance
Data science is a multidisciplinary field that uses scientific methods, processes, algorithms, and systems to extract knowledge and insights from structured and unstructured data. In the context of insurance, it plays a pivotal role in deciphering complex datasets to uncover patterns, trends, and relationships that traditional analysis might miss, thus enabling more informed decision-making [9].
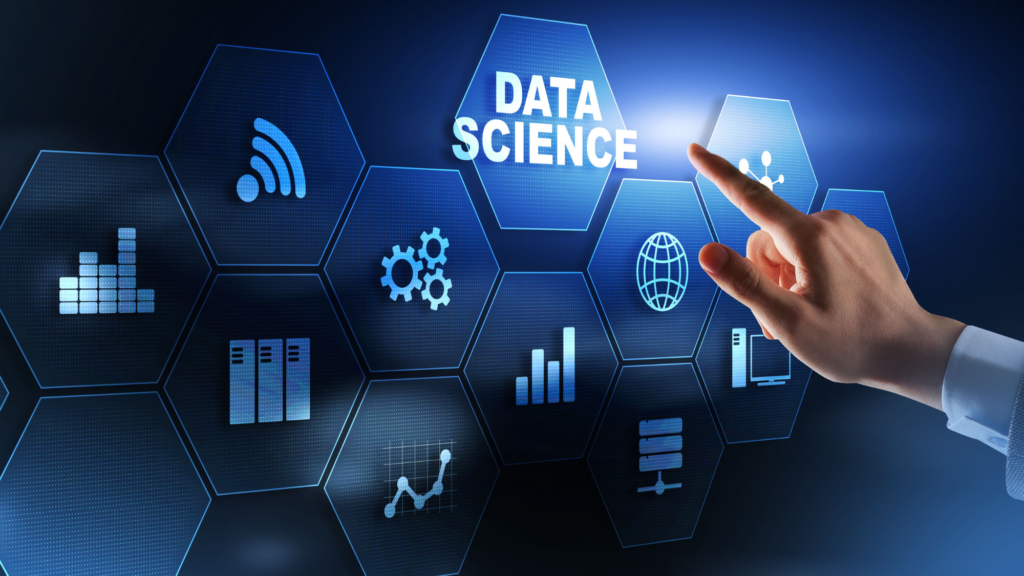
Data science Applications in Insurance:
Applications of data science are numerous and revolutionary in the insurance sector. By using previous data to predict future claims, predictive modeling helps insurers better plan financially and manage risk. By dividing their client base into groups based on shared traits or habits, insurers can better target their offerings with more relevant and appealing products and services. This process is known as customer segmentation. By using individual data points to generate more precise risk profiles, personalized risk assessment enables more equitable and individualized premium pricing. When used in tandem, these apps improve profitability, customer satisfaction, and operational efficiency by facilitating more accurate risk management, product creation, and marketing strategies [10].
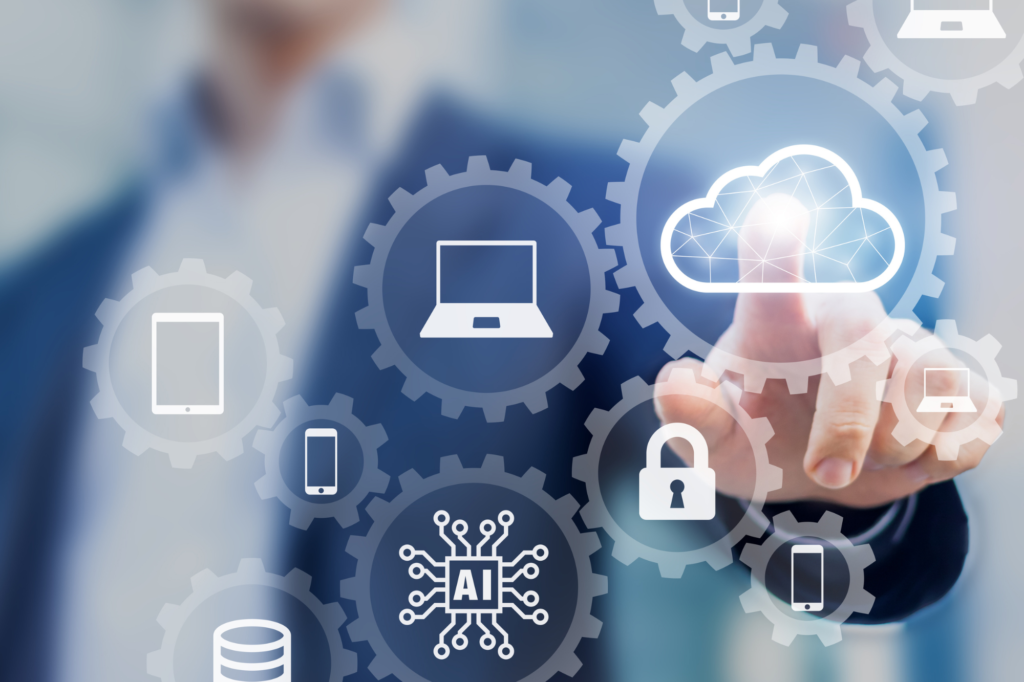
Section 3: Benefits of Data Science for Vehicle Insurance
Precision Targeting:
By applying predictive analytics, data science greatly improves auto insurance firms’ capacity to more accurately target prospective clients. With this method, enormous volumes of data are analyzed to find trends and more accurately forecast client preferences and individual risk profiles. Insurers may thus better target their marketing campaigns and product offers to the unique requirements of various client categories, increasing engagement and conversion rates [11].
Dynamic Pricing Models:
With the aid of data science and dynamic pricing models, insurers can modify rates in response to a thorough examination of each policyholder’s unique risk profile and behavioral patterns. This detailed approach to pricing ensures that premiums are more closely aligned with the real risk that each policyholder represents by accounting for factors like driving habits, claims history, and even lifestyle decisions. Customers are therefore more likely to believe that their insurance costs are reasonable and customized for their particular situation, which can promote a feeling of justice and trust [12].
Customer Satisfaction and Loyalty:
The personalized services and fair pricing strategies enabled by data science contribute significantly to customer satisfaction and loyalty. When customers feel that their insurance provider understands their needs and offers pricing that reflects their individual risk, they are more likely to remain with the company. This personalized approach, coupled with predictive models that ensure customers are offered the right products at the right time, enhances the overall customer experience, thereby increasing loyalty and reducing churn rates [13].
Section 4: Implementing Data Science in Vehicle Insurance
Challenges and Considerations:
Integrating data science into vehicle insurance comes with its set of challenges, notably fractured data and legacy systems which hinder the effective use of data analytics. Companies may not be able to fully utilize data due to these outdated systems, which makes it challenging to draw insightful conclusions and take decisive action [14]. Furthermore, because insurance companies handle sensitive personal and financial data, protecting data privacy and security is a major problem. The market needs experts who can not only comprehend data but also analyze it in the context of insurance, which makes the demand for qualified data scientists difficult to meet .
Strategies for Success:
There are various approaches that can be used to go above these barriers. To start, improving data quality and making sure that data can be used for analytics and AI applications successfully need investment in technology to update legacy systems [4]. To keep client confidence and stay out of legal trouble, it’s also critical to ensure that you’re following data protection rules. This entails putting strong data security measures in place and using open data handling procedures. Furthermore, fostering a data-driven culture within the company might help data science integration go more smoothly. In order to create an atmosphere where data-driven decision-making is respected and promoted, this entails not just employing qualified analysts but also giving resources and training to current employees [15].
FAQs
Q: How does data science improve customer experience in vehicle insurance? A: Data science enables more personalized services, accurate policy pricing, and faster claims processing, improving overall customer satisfaction.
Q: Can data science in vehicle insurance invade privacy? A: While data science relies on extensive data, reputable insurers prioritize data security and privacy, adhering to strict regulations to protect client information.
Q: Are data-driven insurance policies more expensive? A: Not necessarily. Data-driven policies are often more accurately priced based on individual risk, which can lead to savings for safe and responsible drivers.
Q: How can customers benefit from telematics-based insurance policies? A: Customers can benefit from potential premium reductions, personalized feedback on driving behavior, and enhanced safety features such as automatic emergency assistance.
Conclusion
In summary, the adoption of data science by the auto insurance sector signifies a paradigm change in favor of dynamic pricing models, more precise risk assessment, and individualized interaction with consumers. Insurance companies can analyze large datasets to find patterns and insights that are missed by conventional methods by utilizing sophisticated analytics and machine learning.
This makes it possible to create insurance plans that support more equitable pricing methods while also reflecting the unique risk profiles of their members. Precision targeting and customized services that follow improve client happiness and build loyalty and trust. Additionally, by implementing data science approaches, insurance firms may better negotiate the intricacies of contemporary risk assessment, increasing operational effectiveness and profitability.
As we move forward, the continued evolution and ethical application of data science in insurance will undoubtedly play a pivotal role in reshaping industry standards and expectations, making it an indispensable asset in the insurers’ toolkit.
Vehicle insurance companies are at a pivotal juncture where embracing data science can significantly transform their operations and competitive stance. The integration of predictive analytics into the insurance process not only enhances risk assessment capabilities but also opens avenues for creating personalized customer experiences. This strategic adoption of data analytics is crucial for tapping into insights across the insurance value chain, leading to more informed decision-making and innovative product development .
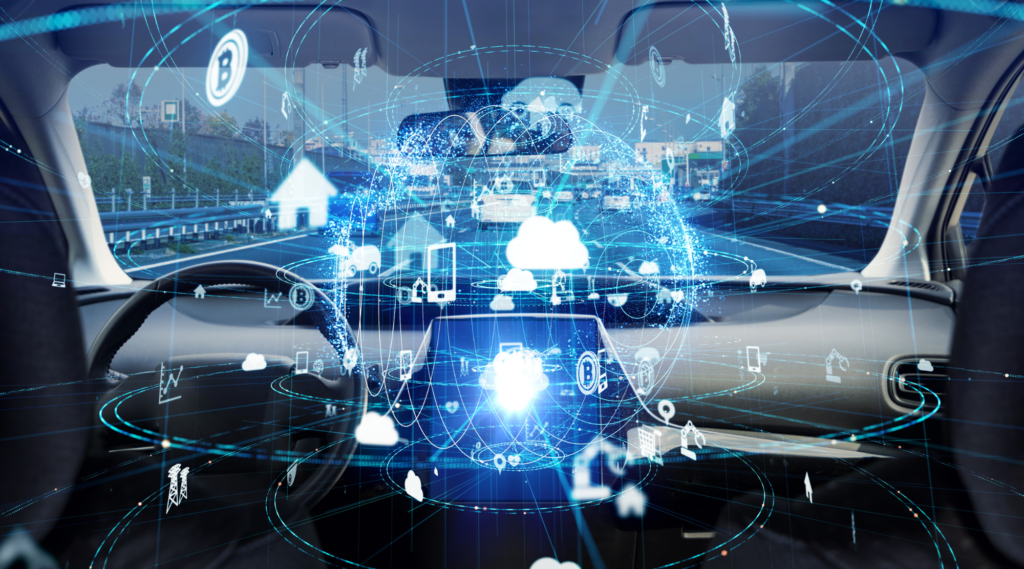
By leveraging data analytics, insurance companies can shift from traditional, static underwriting methods to dynamic, real-time risk assessment, enabling more accurate pricing and product offerings that reflect the individual risk profiles and preferences of customers. The future of car insurance is being shaped by big data, allowing companies to draw on diverse and continuously updating data sources for more precise actuarial calculations .